Special Edition Blog Series on PhD Abstracts (Part III)
This post is a continuation of the blog post series on PhD abstracts. You can find the first part of the series here.
Mitisha Gaur: Re-Imagining the Interplay Between Technical Standards, Compliances and Legal Requirements in AI Systems Employed in Adjudication Environments Affecting Individual Rights
The doctoral thesis investigates the use of AI technology in automated decision making systems (ADMS) and subsequent application of these ADMS within Public Authorities as Automated Governance systems in their capacity as aides for the dispensing of public services and conducting investigations pertaining to taxation and welfare benefits fraud. The thesis identifies Automated Governance systems as a sociotechnical system comprising three primary elements- social (workforce, users), technical (AI systems and databases) and organisational (Public Authorities and their internal culture).
Fuelled by the sociotechnical understanding of automated governance systems, the thesis’ investigation is conducted through three primary angles, Transparency, Human Oversight and Algorithmic Accountability and their effect on the development, deployment and subsequent use of the Automated Governance systems. Further, the thesis investigates five primary case studies against the policy background of the EU’s HLEG Ethics guidelines for AI systems and the regulatory backdrop of the AI Act (and on occasion the GDPR).
Finally, the thesis concludes with observed gaps in the ethical and regulatory governance of Automated Governance systems and recommends core areas of action such as the need to ensure adequate agency for the decision-subjects of the AI systems, the importance of enforcing contextual clarity within AI Systems deployed in a high risk scenario such as Automated Governance and advocates for strict ex-ante and ex-post requirements for the developers and deployers of Automated Governance systems.
Maciej Zuziak: Threat Detection and Privacy Risk Quantification in Collaborative Learning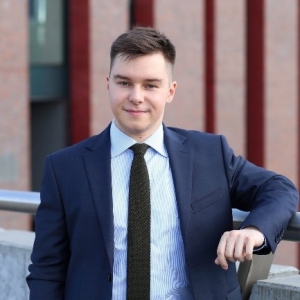
This thesis compiles research on the brink of privacy, federated learning and data governance to answer numerous issues that concern the functioning of decentralised learning systems. The first chapters introduce an array of issues connected with European data governance, followed by an introduction of Data Collaboratives – a concept that is built upon common management problems and serves as a generalization of numerous approaches to collaborative learning that have been discussed over the last years. The subsequent work presents the results of the experiments conducted on the selected problems that may arise in collaborative learning scenarios, mainly concerning threat detection, clients’ marginal contribution quantification and assessment of re-identification attacks’ risk. It formalizes the problem of marginal problem contribution, introducing a formal notion of Aggregation Masks and Collaborative Contribution Function that generalizes many already existing approaches such as Shaple Value. In relation to that, it presents an alternative solution to that problem in the form of Alpha-Amplification functions. The contribution analysis is tied back to threat detection, as the experimental section explores using Alpha Amplification as an experimental method of identifying possible threats in the pool of learners. The formal privacy issues are explored in two chapters dedicated to spoofing attacks in Collaborative Learning and the correlation between the former and membership inference attacks, as the lack thereof would imply that similar (deletion-based) metrics would be safe to employ in the Collaborative Learning scenario. The last chapter is dedicated to the selected compliance issues that may arise in the previously presented scenarios, especially those concerning the hard memorization of the models and the consent withdrawal after training completion.